International Journal of Clinical Cardiology
Discriminant Analysis of Heart Rate Variability after Electrical Cardioversion Predicts Atrial Fibrillation Recurrence
Donatella B, Angela V, Emilia I, Kristian E1, Claudia C1, Rita SA1, Francesco F and Riccardo F*
Clinical Physiology - Bio magnetism Centre, Catholic University of Sacred Heart, Italy
*Corresponding author: Prof. Fenici Riccardo, MD, Clinical Physiology-Biomagnetism Center, Catholic University of Sacred Heart, Largo Agostino Gemelli, 8, 00168 Rome, Italy, Tel: +39 063051193; Fax: +39 063051343; E-mail: feniciri@rm.unicatt.it; dbrisinda@libero.it
Int J Clin Cardiol, IJCC-1-010, (Volume 1, Issue 2), Research Article; ISSN: 2378-2951
Received: October 22, 2014 | Accepted: November 22, 2014 | Published: November 25, 2014
Citation: Donatella B, Angela V, Emilia I, Kristian E, Claudia C, et al. (2014) Discriminant Analysis of Heart Rate Variability after Electrical Cardioversion Predicts Atrial Fibrillation Recurrence. Int J Clin Cardiol 1:010. 10.23937/2378-2951/1410010
Copyright: © 2014 Donatella B, et al. This is an open-access article distributed under the terms of the Creative Commons Attribution License, which permits unrestricted use, distribution, and reproduction in any medium, provided the original author and source are credited.
Abstract
Aims: Cardiac autonomic modulation (CAM) may be pivotal for atrial fibrillation (AF) occurrence and affect early recurrence (ER) after electrical cardioversion (EC). Previous studies investigating linear (L) heart rate variability (HRV) after EC have given conflicting results about which CAM pattern favours ER. This study aimed at evaluating if non-linear (NL) HRV analysis (HRVa) could provide better accuracy in predicting ER.
Methods: 36 patients, 16 under antiarrhythmic drugs (AAD), were enrolled after EC for persistent AF. Stable sinus rhythm (SR) was obtained in 34. HRVa was performed, with L and NL methods, from five-minutes time-segments selected within the first hour after EC and the subsequent 24 (daily activity and sleep). Discriminant Analysis (DA) was used to evaluate which parameters were efficient in predicting ER of AF.
Results: When comparing 14/34 patients with AF ER (Group1) with twenty who maintained SR (Group2), no significant difference were found immediately after EC and during daily activity. However, sympathetic prevalence was found in Group1 during sleep. At DA, only NL SampEn predicted AF ER (accuracy: 73.5%). In 18 patients without AAD pre-treatment, higher sympathetic modulation during daily activity and vagal prevalence during sleep were found in patients without AF ER (p< 0.05). DA during sleep predicted AF recurrence with 83.3% and 100% accuracy when using L and NL parameters, respectively.
Conclusions: During the first 24 hours after EC, CAM is different in patients with and without AF ER. NL HRVa exploring the complexity of CAM of HRV predicts AF ER with better accuracy.
Introduction
Atrial fibrillation (AF) is the most common arrhythmia, whose estimated prevalence is approximately 1.5-2% of the general population (average age 75-85 years old) and is expected to affect approximately 30 million North Americans and Europeans by 2050 [1,2]. It is associated with a five-fold risk of stroke, a three-fold incidence of congestive heart failure, higher mortality and hospitalization [1]. Sinus rhythm (SR) restoration with electrical cardio version (EC) is one of the treatment options, but available data evidenced that AF relapses in 40-60% of cases (with prevalence of recurrence within two weeks after EC), even with the presence of appropriate anti-arrhythmic therapy [3,4]. Shortening of the atrial refractory period [5], electrical remodelling [6,7], structural/anatomical remodeling of the atrial cardiomyocytes [8] and modifications in autonomic nervous system (ANS) pattern [9-12] have been considered responsible for recurrence of AF.
In 1994, Coumel [13] introduced the concepts of "vagal AF" preferentially observed in the absence of structural cardiac abnormalities (because vagal influences are predominant in normal hearts) and "sympathetic mediated AF", observed in the presence of heart disease that might provoke a vagal withdrawal.
Since 1996, Heart Rate Variability analysis (HRVa) [14] has been applied to evaluate the ANS modulation also in patients with AF. In particular, on the basis of spectral analysis of the HRV, used to predict the onset of paroxysmal atrial fibrillation (PAF) events [15-18], a classification of three types of spectral changes preceding PAF onset has been proposed, with increase in the LF band and decrease in the HF band (type A), decrease in the LF band and increase in the HF band (type B), and sometimes simultaneous increase in both LF and HF bands (type C) [19]. Instead, relatively few and conflicting results have been reported on the predictive value of HRVa to identify the autonomic pattern favouring AF recurrence after EC [5,12,20]. In fact, either sympathetic hypertone with vagal withdrawal [4,20] or parasympathetic prevalence [5,12] have been suggested as autonomic patterns favouring AF recurrence. One possible reason of such uncertainty could be that conventionally used time-domain (TD) and frequency-domain (FD) parameters of HRV are not always suitable for analysis because of the non-stationarity characteristic of the ECG recordings and the presence of nonlinear phenomena in the physiological signal's parameter variability. HRVa by the Poincare plot [21] and quantification of the complexity of the heart rate using sample entropy (SampEn) [22] have been used to evidence cardiac autonomic neuropathy in diabetic patients [23]. The analysis of the complexity of HRV dynamics identified a significant decrease of SampEn and approximate entropy (ApEn) before PAF events [19,24-26], however, to the best of our knowledge, HRV complexity to predict AF recurrence after EC was so far evaluated in few studies only [27,28]. Therefore, in this study HRVa was carried out not only in the TD and FD, but also with non-linear (NL) methods, with the aim to assess the predictive value of complexity parameters in identifying patients prone to early recurrence of AF after EC.
Methods
Patients: 36 consecutive patients (26 males and 10 females, mean age 67.5 + 9. 2 years) with persistent AF (mean duration 8.18 + 15.3 months) were enrolled. Restoration of stable SR was obtained in 34/36 of subjects (94.4%) that were included in the study. Clinical characteristic of the study population are summarized in Table 1.
Table 1: Clinical and echocardiographical characteristics of study population with
(Group 1) and without (Group 2) AF recurrence.
View Table 1
Clinical and pharmacological history, physical examination, 12-lead electrocardiogram (ECG) and trans-thoracic two-dimensional imaging and Doppler echocardiography were performed in all patients before EC. All subjects were under oral anticoagulant therapy, with the INR value in therapeutic range (2-3) for at least 4 weeks before EC.
At the moment of the EC procedure 16 patients were under pharmacological treatment with anti-arrhythmic drugs (AAD) (i.e. Flecainide, Propafenone, or Amiodaron). In 10 patients needing beta-blockers for appropriate rate-control, the drugs were withdrawn at least twenty-four hours before the EC procedure. The study was approved by the local Ethics Committee and was performed in accordance with the ethical standards of the 1964 Declaration of Helsinki. All patients gave informed consent prior to EC and their inclusion in the study.
Electrical cardioversion and follow-up: A 12-lead ECG was recorded before EC, in order to confirm persistent AF. EC was performed under cardio-respiratory monitoring, with slight anaesthesia (with 1 mg/kg intravenous Propofol) and spontaneous breathing.
External biphasic DC shock was delivered with an M Series cardioverter (Zoll Medical, Burlington, MA, USA). Delivered energy ranged between 75 and 150 Joule (mean 109.2 + 11.6). A second DC shock was delivered in five patients, who had relapsed in AF almost immediately after the first one. Stable SR restoration was obtained in 34 subjects (94.4%). No complication occurred, nor was respiratory assistance needed during the procedure.
12-lead ECG was continuously recorded during the procedure with telemetric monitoring (Surveyor Central - Mortara Instruments Inc, Milwaukee, WI, USA ) and thereafter for the subsequent 24 hours with 12-lead H-Scribe Holter monitoring (H-Scribe-Mortara Instruments Inc, Milwaukee, WI, USA ), with High Fidelity card (sampling frequency: 1 KHz).
At the end of the 24 h of Holter monitoring, prophylactic AAD treatment was continued in 16 patients and started in other 10.
A follow-up 12-lead ECG was scheduled at 5 and 15 days after EC, or recorded as soon as possible if symptomatic palpitations occurred. The average follow-up after EC was 23.5 + 11.3 months.
Early recurrence (ER) was arbitrarily defined as relapse of AF within ninety days after EC and SR stability was defined as maintenance for at least twelve months.
HRV analysis: Short-term (i.e. from standard 5-minutes time segments of the tachogram) quantitative HRVa was performed according to the guidelines of the European Society of Cardiology and the North American Society of Pacing and Electrophysiology [14,29,30]. Briefly, first raw ECG data were extracted from the telemetric and/or Holter recording with a custom software routine and edited to manually remove technical artifacts and/or physiological artifacts. The fraction of total RR intervals labeled as normal-to-normal (NN) intervals was used as a measure of data reliability, with the purpose to exclude records with a ratio less than a 90% threshold. Then a further editing was performed by visual analysis of the tachogram and of corresponding ECG, with manual correction of possible residual artifacts. Finally, HRV parameters were calculated in the TD, FD and with NL methods (Table 2) using the Kubios HRV software (version 2.1) [31]. Since "stationarity" is crucial for data analysis, we tried to optimize the selection of the 5-minute time-segments used for calculation by looking for: 1) the best coherence among spectral output obtained with the Fast Fourier Transform (FFT) and autoregressive (AR) models and 2) the highest possible "stationarity" of the RR signal (defined not only as the absence of arrhythmia and of any kind of artifacts in visual analysis of corresponding ECG recordings, but also on the basis of a visual check of the stability of spectral pattern within each 5-minute segment used for quantitative analysis by manually scanning it with a 30-seconds window). Moreover an advanced detrending procedure (i.e. the "smoothness priors regularization" [32], was used to remove slow non stationarities in HRV [33].
Table 2: Descriptive statistics of HRV parameters calculated in all patients after electrical cardioversion.
View Table 2
Physiological interpretation of HRV parameters: HRV parameters were calculated in the time-domain, frequency-domain and with NL methods.
According to the literature, in the TD, SDNN was considered an indicator of overall HRV, RMSSD and pNN50% indexes of vagal modulation. The FD [VLF = very low frequency (band < 0.04 Hz); LF = low frequency (band: 0.04 -0.15 Hz); HF = high frequency (band: 0.15 - 0.40 Hz)] parameters, calculated with both the Fast Fourier Transform (FFT) model and with parametric autoregressive (AR) algorithms, were expressed in absolute (ms2), in percent (%) and in normalized unit (n.u.). The HF component is an index of parasympathetic modulation [14,30,34]. The LF component is related to baroreceptor control, and may reflect both sympathetic and parasympathetic activity [14,30,34]. Although its physiological interpretation is still controversial [35], it is usually accepted as an index of sympathetic modulation, especially when expressed in normalized units (n.u.) [4,34]. The LF/HF and SDNN/RMSSD [36-38] ratios were used as indices of sympato-vagal interaction [4,34,39]. The VLF component is related to humoral factors as thermoregulatory process and renin-angiotensin system [39,40].
Among NL methods, the Poincare plot is a NL two-dimensional visualization tool for dynamic systems. The width (SD1) and the length (SD2) of the Poincare plot represent short and long-term HRV, respectively. A correlation was previously described between HF power and SD1 [21,23,41], as well as between LF and HF power and SD2 [21,41] SD1/SD2 represents a ratio between short- and long-term variability, and low values are correlated with ANS dysfunction [21].
As concern Detrended Fluctuation Analysis (DFA) parameters (a1, a2), the correlations are separated in short- and long-term HRV fluctuations. The short-term fluctuations are characterized by the slope a1 inside the range 4 < n < 16, while long term fluctuations are characterized by the slope a2, whose range is 16 < n < 64.
The correlation dimension (d2) is another way to measure the complexity of a time series. It gives information on the minimum number of dynamic variables needed to model the underlying system [42]. Recurrence Plot (RP) analysis (RPlmean, RPlmax, RPrec, RPadet, RPshen) is an advanced technique of NL analysis that shows when the time series visits the same region of phase space. It is a two-dimensional representation technique that defines whether a system is periodic or chaotic [43].
Non-linear Entropy (ApEn, SampEn) is a quantitative index for the evaluation of the unpredictability and complexity of the heart rate. The advantage of SampEn is its independence on time-series length, thus it can be computed from shorter HRV records [22]. A time series containing many repetitive patterns has a relatively small SampEn, while a more complex (i.e., less predictable) process has a relatively high SampEn [26]. SampEn values are physiologically higher during sleep than during daily activity. Low SampEn values indicate an increase in regularity and a decrease in complexity of HRV and suggest impairment of the cardiovascular autonomic control system [22,23].
Statistical analysis: HRVa was carried out first on the whole population divided in Group 1 (14 patients, with early AF recurrence) and Group 2 (20 patients, without AF recurrence). However, taking into account that 16 patients were pre-treated with AAD at the moment of EC, a second analysis was separately carried out with the data of the 18 untreated (Group 1a and Group 2a) only, to rule out possible pharmacological effects of AAD on the assessment of autonomic modulation.
All statistical calculations were performed with SPSS software, version 13.0 (SPSS Inc., Chicago, Illinois) [44]. Continuous variables are presented as mean + standard deviation (SD), unless otherwise indicated. The comparison of continuous variables was performed with the Student t (considering a significant p < 0.05) [45,46].
To determine whether there were statistically differences in the features extracted from patients with or without AF recurrence, the Mann-Whitney U test or by unpaired Student t-test were used for continuous variables, and the chi-square or by Fisher test exact test for categorical variables.
All of the extracted features were also tested with ANOVA (analysis of variance) patients with AF recurrence episodes and patients remaining in stable SR. p-values less than 0.05 were considered statistically significant.
Discriminant Analysis (DA) was used to evaluate if HRV parameters were adequate to provide a separation between patients with AF early recurrence and those without. DA searches for linear combinations of the input features that can provide an adequate separation between the two patient groups. The discriminant functions used by DA are built up as a linear combination of the variables that seek to maximize the differences between the groups [47]. The classification accuracy of the method is defined as the ability to discriminate between the two investigated groups.
Results
Of the 36 patients initially recruited, immediate relapse of AF occurred in two. Out of 34 patients in whom stable sinus rhythm was obtained, AF recurred in 14 (41%) (Group 1), within 3-9 days after EC (mean 5.8 + 2.8 days) in four, within 15-30 days (mean 23.8 + 7.5 days) in seven, and within 90 days in three. 20 patients remained in stable SR (Group 2).
No statistically significant differences were found between the two groups in mean age, in clinical history, underlying heart disease and echocardiographic parameters (Table 1), with the exception of AF duration in months, which was longer in Group 1 (14.5 + 22.6) compared with Group 2 (3.7 + 3.5, p < 0.05).
HRV analysis in all patients: At the first hour after EC, no significant difference of HRV parameters was found between Group 1 and Group 2.
During daily activity, only VLF power (in %) was significantly higher in Group 2 (p< 0.05) (Table 2).
During night sleep, more significant differences were found. In fact, the physiological prevalence of vagal tone was maintained in Group 2 patients [lower SDNN/RMSSD (p< 0.05), higher SD1/SD2 (p< 0.05), and higher SampEn (p< 0.05)] but not in Group 1 patients, in which higher values of RP parameters (RPadet and RPrec) were found (Table 2). However at DA only SampEn during sleep was efficient in predicting ER of AF (predictive accuracy: 73.5%).
An example of typical patterns of linear and NL HRV analysis of one Group 1 and of one Group 2 patients is shown in Figure 1.
Figure 1: Examples of typical HRV patterns and values of one patient with (Group 1) and one without AF recurrence (Group 2). During sleep, dominant HF spectral
component (in yellow), higher SD1/SD2 ratio and lower LF/HF ratio (0.14), all indices of vagal prevalence, characterize the Group 2 patient. Instead, sympathetic
tone is dominant [higher LF component (in blue) and higher LF/HF (14.3)] in the Group 1 patient. Also entropy values are lower in the patient with AF recurrence.
a) tachogram; b) spectral analysis; c) quantitative FD results calculated with AR (ARMA) model; d) quantitative results of non-linear analysis; e) examples of
Poincare plot. (PSD: Power spectral density).
View Figure1
Within Group 1 patients, HRV analysis was not predictive of the timing of AF recurrence (no difference was found in autonomic modulation between patients relapsing in AF within 3-9 days and those who had AF occurring after thirty or more days).
HRV analysis in the subgroup of patients not pre-treated with AAD
At the first hour after EC, no significant difference of HRV parameters was found in the subgroups of patients who were not pre-treated with AAD before EC (Group 1a and Group 2a).
During daily activity, only RPlmax and ApEn (p< 0.05) were significantly different between the two groups (higher in Group 2a) (Table 3). DA analysis of linear parameters predicted AF (if F1 < 0 in the formula: F1=-0.01 x HFms2fft -0.12 x LF n.u. fft +1.11 x LF/HF fft +0.07 x HF%AR +0.22 x LF n.u.AR -0.97 x LF/HFAR -8.58) with relatively low (72.2%) accuracy. However predictive accuracy improved to 88.9% with the combination of NL parameters in the formula: F2 = -5.23 x SD1/SD2 +16.41 x ApEn -15.06 (Table 4A).
Table 3: Descriptive statistics of HRV parameters of patients without AAD pre-tratment before electrical cardioversion.
View Table 3
Table 4: Discriminant analysis in 18 patients without AAD pre-treatment (Group 1a vs Group 2a) . during daily activity (4A) and night sleep (4B). Performance of the
classification rules based on single HRV parameter and combination of parameters.
View Table 4
Differences between Group 1a and Group 2a were more significant during sleep. In fact in Group 2a a prevalence of vagal tone was evidenced by both linear [lower SDNN/RMSSD, higher HF power (n.u. and in %), lower LF power (n.u.), lower LF/HF ratio] and NL analysis [higher SD1/SD2 ratio, higher SampEn and ApEn, lower RPrec and RPadet] (p< 0.05) (Table 4). DA, applied to linear parameters (combined in the formula: F1=1.76 x SDNN/RMSSD + 0.01 x VLF ms2 - 0.03 x HF% + 0.01 x LF n.u. -2.31), predicted AF recurrence with 83.3% accuracy. The predictive accuracy improved to 100% with the combination of NL parameters in the formula: F2=12.71 x SD1/SD2 + 6.44 x DFA a1 -19.50 x ApEn -7.8 x SampEn +20.35 (AF recurrence if F2 > 0) (Table 4B).
Discussion
AF recurrence after EC ranges between 40 and 60% 4 and may depends on multiple factors such as electrical [6,7] and structural/anatomical remodeling [8] of the atrial cardiomyocytes as well as abnormal ANS modulation [4,9-12]. In fact, non-uniform autonomic innervation of the atria has been demonstrated by Alessi in animal models of AF [48] and asymmetric distribution of vagal innervation could determinate dispersion of atrial electrical refractoriness responsible of the increased vulnerability of the atria [5]. Previous studies evaluating spectral HRV parameters immediately (from 30 minutes to 1 hour) after EC of persistent AF, demonstrated that increased vagal tone is predominant in the early phases after SR restoration [5] as well as on the second post-cardioversion day [12], and in another study it has been also reported that an increased HF component during the first week after EC was the most powerful predictor of AF recurrence [27].
In contrast, Lombardi et al., performing short-term spectral HRVa, at about 4 to 5 hours after SR restoration, to predict AF recurrence at 12 to 15 days, evidenced that greater incidence of early AF recurrence occurred in patients with signs of enhanced sympathetic and reduced vagal modulation of the sinus node [4]. Similar findings were reported also by Michelucci et al. [49].
More recently, NL analytic methods of AF waves were used to predict effectiveness of a shock in EC [50,51] and AF early recurrence [52], evidencing an higher SampEn (or increase of complexity) of AF waves in patients who relapsed [51,52]. This is not in contrast and must not be confused with previous results that indicate lower HRV SampEn as an index of impairment of the cardiovascular control system and risk marker of cardiac autonomic neuropathy in diabetes [23].
Such contrasting results, which suggest that a more complex scenario of different autonomic patterns could favour early or late AF recurrence 5, motivated this study aiming to understand whether simultaneous evaluation of HRV and of its complexity, with NL methods, could improve the accuracy to predict AF recurrence after EC.
As autonomic imbalance can sometime become evident only in specific conditions, HRV analysis was carried out not only in the early phase after EC, but also during normal daily activity and night sleep, along the subsequent twenty-four hours.
Acknowledging possible effects of AAD pre-treatment on the outcome of EC as well as on the results of HRV analysis, the comparison was carried out not only between the total number of patients in which AF relapses (Group 1) vs patients in which AF no relapses (Group 2), but also in the subgroup of eighteen patients without AAD pre-treatment (Group 1a and Group 2a) at the moment of EC. Instead, separate analysis of the subgroup of patients under AAD was not performed because the number of patients treated with each drug (Flecainide, Propafenone or Amiodaron), was too small to allow any statistical evaluation of the results.
As expected, when comparing all patients, without caring about possible interference of ongoing AAD treatment, the accuracy of HRV analysis to predict ER of AF was relatively low (73.5%) and limited to the findings obtained during night sleep, because practically no significant difference of TD, FD and NL features was found between Group 1 and Group 2 at the first hour after EC and under daily activity. However, depressed vagal modulation and a prevalence of sympathetic modulation during night sleep characterized Group 1 patients as evidenced by significantly higher values of SDNN/RMSSD, by lower values of SD1/SD2 ratio, by lower value of SampEn and by higher values of RP parameters (RPrec, RPadet) (p< 0.05) (Table 2). This finding suggests that impairment of vagal tone during sleep is associated with AF relapse after EC, and is consistent with previous observations that vagal withdrawal predisposes to cardiac arrhythmias [53,54] as well as to progression of chronic heart failure, ischemic heart disease and hypertension [55,56].
HRV analysis carried out in the subgroup of eighteen patients without anti-arrhythmic treatment at the moment of EC was more sensitive in differentiating between patients with and without AF recurrence. A larger number of linear and NL HRV parameters during night sleep, and two NL parameters (RPlmax and ApEn) also during daily activity were significantly different between the two groups (Table 3). Substantial prevalence of sympathetic modulation and lower HRV complexity during sleep (higher SDNN/RMSSD ratio, lower HF power, higher LF power and higher LF/HF ratio, lower values of ApEn and SampEn, lower SD1/SD2 ratio) were confirmed as significant markers of risk for ER of AF (Table 3). Thus unbalance of cardiac autonomic modulation favoring sympathetic prevalence (and depression of vagal tone) during night sleep may predispose to AF recurrence [4,49].
Instead, in Group 1a patients early AF recurrence was correlated with a tendency of prevalent vagal modulation during daily activity, evidenced by lower SDNN/RMSSD ratio, higher HF component, lower LF component and lower LF/HF ratio, which however did not reach statistical significance. This finding is in agreement with previous results of Vikman et al. [27] that described an increased HF component during the first week after EC, and considered this parameter as the most powerful predictor of AF recurrence.
DA analysis was applied to define which HRV features could provide the best differentiation between patients with and without AF recurrence. The predictive accuracy of linear HRV parameters calculated during daily activity and during sleep was 72.2% and 83.3 respectively (Tables 4A,4B). If DA was applied to NL HRV parameters, calculated in the same conditions, the predictive accuracy improved to 88.9% and 100% respectively.
Thus NL analysis provides better predictive accuracy and seems to be much more reliable and stable in both condition (daily activity and sleep), probably because it is less affected by "non-stationarity" that is typical of the complexity of biological systems [33,57]. In fact, NL measures differ from linear HRV analytic methods because they are not designed to assess the magnitude of variability but rather the quality, scaling, and correlation properties of the HR dynamics [58]. In contrast with the findings of Vikman [27] our Group 2a of patients, in which AF did not relapse in the absence of AAD treatment, NL HRV analysis evidenced higher level of complexity and entropy that are indices of healthy cardiac autonomic modulation that could be protective against AF recurrence.
Limitations of the study: None of TD and FD HRV parameters was useful in differentiating patients with early or late AF recurrence, as also reported by Bertaglia et al. [5], but in contrast with the results of other authors [4,5,12,20]. This negative finding could be due to the limited number of analyzed cardioversions, although the overall number of patients was enough to obtain 80% of power on statistical analyses with "a" level of 0.05. For the same reason in spite of the significant predictive accuracy obtained in this study with DA of a combination of NL parameters, a wider data set will be required in order to differentiate the outcome of subgroups of patients undergoing EC with and without AAD pre-treatment or maintained in AAD therapy after sinus rhythm restoration and to confirm the robustness of the method.
Conclusions
Although NL HRV parameters have been previously used to predict the onset of AF or its recurrence after EC [24-28], this study aimed to a more comprehensive understanding of cardiac autonomic modulation occurring in the first twenty-four hours following EC, to identify efficient predictors of early recurrence of AF by discriminant analysis of a larger number of HRV parameters simultaneously evaluated with linear and non-linear methods.
Our results evidenced that AF relapsed more frequently in patients who presented lower HRV complexity and entropy in the first twenty-four hours after cardioversion, as well as prevalence of sympathetic tone and lower vagal modulation during sleep in the night after SR restoration.
Therefore routine evaluation of the complexity of autonomic modulation of heart rate after EC might provide information relevant to predict long-term efficacy of electrical cardioversion and to select appropriate AAD treatment to prevent AF recurrence.
References
-
Camm AJ, Lip GY, De Caterina R, Savelieva I, Atar D, et al. (2012) 2012 focused update of the ESC Guidelines for the management of atrial fibrillation. An update of the 2010 ESC Guidelines for the management of atrial fibrillation Developed with the special contribution of the European Heart Rhythm Association. Eur Heart J 33: 2719-2747.
-
Hoogstra-Berends F, Meijering RA, Zhang D, Heeres A, Loen L, et al. (2012) Heat shock protein-inducing compounds as therapeutics to restore proteostasis in atrial fibrillation.Trends Cardiovasc Med 22: 62-68.
-
Zipes DP (1997) Atrial fibrillation. A tachycardia-induced atrial cardiomyopathy.Circulation 95: 562-564.
-
Lombardi F, Colombo A, Basilico B, Ravaglia R, Garbin M, et al. (2001) Heart rate variability and early recurrence of atrial fibrillation after electrical cardioversion.J Am CollCardiol 37: 157-162.
-
Bertaglia E, Zoppo F, Bonanno C, Pellizzari N, Frigato N, et al. (2005) Autonomic modulation of the sinus node following electrical cardioversion of persistent atrial fibrillation: relation with early recurrence. Int J Cardiol 102: 219-223.
-
Tieleman RG, Van Gelder IC, Crijns HJ, De Kam PJ, Van Den Berg MP, et al. (1998) Early recurrences of atrial fibrillation after electrical cardioversion: a result of fibrillation-induced electrical remodelling of the atria? J Am CollCardiol 31: 167-173.
-
Wijffels MC, Kirchhof CJ, Dorland R, Allessie MA (1995) Atrial fibrillation begets atrial fibrillation. A study in awake chronically instrumented goats.Circulation 92: 1954-1968.
-
Ausma J, Wijffels M, Thoné F, Wouters L, Allessie M, et al. (1997) Structural changes of atrial myocardium due to sustained atrial fibrillation in the goat.Circulation 96: 3157-3163.
-
KILLIP T, GAULT JH (1965) MODE OF ONSET OF ATRIAL FIBRILLATION IN MAN.Am Heart J 70: 172-179.
-
Waxman MB, Wald RW, Cameron D (1983) Interactions between the autonomic nervous system and tachycardias in man.CardiolClin 1: 143-185.
-
Blaauw Y, Tieleman RG, Brouwer J, Van Den Berg, De Kam PJ, et al. (1999) Tachycardia induced electrical remodeling of the atria and the autonomic nervous system in goats.Pacing ClinElectrophysiol 22: 1656-1667.
-
Kanoupakis EM, Manios EG, Mavrakis HE, Kaleboubas MD, Parthenakis FI, et al. (2000) Relation of autonomic modulation to recurrence of atrial fibrillation following cardioversion.Am J Cardiol 86: 954-958.
-
Coumel P (1994) Paroxysmal atrial fibrillation: a disorder of autonomic tone?Eur Heart J 15 Suppl A: 9-16.
-
(1996) Heart rate variability: Standards measurement, physiological interpretation, and clinical use. Task force of the European Society of Cardiology and the North American Society of pacing and Electrophysiology. Circulation 17: 354-381.
-
Bettoni M, Zimmermann M (2002) Autonomic tone variations before the onset of paroxysmal atrial fibrillation.Circulation 105: 2753-2759.
-
Amar D, Zhang H, Miodownik S, Kadish AH (2003) Competing autonomic mechanisms precede the onset of postoperative atrial fibrillation.J Am CollCardiol 42: 1262-1268.
-
Tomita T, Takei M, Saikawa Y, Hanaoka T, Uchikawa S, et al. (2003) Role of autonomic tone in the initiation and termination of paroxysmal atrial fibrillation in patients without structural heart disease. J CardiovascElectrophysiol14: 559-564.
-
Fioranelli M, Piccoli M, Mileto GM, Sgreccia F, Azzolini P, et al. (1999) Analysis of heart rate variability five minutes before the onset of paroxysmal atrial fibrillation.Pacing ClinElectrophysiol 22: 743-749.
-
Chesnokov YV (2008) Complexity and spectral analysis of the heart rate variability dynamics for distant prediction of paroxysmal atrial fibrillation with artificial intelligence methods.ArtifIntell Med 43: 151-165.
-
Akyurek O, Diker E, Guldal M, Oral D (2003) Predictive value of heart rate variability for the recurrence of chronic atrial fibrillation after electrical cardioversion.ClinCardiol 26: 196-200.
-
Brennan M, Palaniswami M, Kamen P (2001) Do existing measures of Poincare plot geometry reflect nonlinear features of heart rate variability?IEEE Trans Biomed Eng 48: 1342-1347.
-
Richman JS, Moorman JR (2000) Physiological time-series analysis using approximate entropy and sample entropy.Am J Physiol Heart CircPhysiol 278: 2039-2049.
-
Khandoker AH, Jelinek HF, Palaniswami M (2009) Identifying diabetic patients with cardiac autonomic neuropathy by heart rate complexity analysis.Biomed Eng Online 8: 3.
-
Shin DG, Yoo CS, Yi SH, Bae JH, Kim YJ, et al. (2006) Prediction of paroxysmal atrial fibrillation using nonlinear analysis of the R-R interval dynamics before the spontaneous onset of atrial fibrillation.Circ J 70: 94-99.
-
Vikman S, Makikallio TH, Yli-Mayry S, Pikkujamsa S, Koivisto AM, et al. (1999) Altered complexity and correlation properties of R-R interval dynamics before the spontaneous onset of paroxysmal atrial fibrillation.Circulation 100: 2079-2084.
-
Mohebbi M, Ghassemian H (2012) Prediction of paroxysmal atrial fibrillation based on non-linear analysis and spectrum and bispectrum features of the heart rate variability signal. Comput Methods Programs Biomed 105: 40-49.
-
Vikman S, Makikallio TH, Yli-Mayry S, Nurmi M, Airaksinen KE, et al. (2003) Heart rate variability and recurrence of atrial fibrillation after electrical cardioversion.Ann Med 35: 36-42.
-
Zohar P, Kovacic M, Brezocnik M, Podbregar M (2005) Prediction of maintenance of sinus rhythm after electrical cardioversion of atrial fibrillation by non-deterministic modelling. Europace 7: 500-507.
-
Lombardi F, Stein PK (2011) Origin of heart rate variability and turbulence: an appraisal of autonomic modulation of cardiovascular function.Front Physiol 2: 95.
-
Sztajzel J, Jung M, Bayes de Luna A (2008) Reproducibility and gender-related differences of heart rate variability during all-day activity in young men and women.Ann Noninvasive Electrocardiol 13: 270-277.
-
Tarvainen MP, Niskanen JP, Lipponen JA, Ranta-Aho PO, Karjalainen PA (2014) Kubios HRV--heart rate variability analysis software.Comput Methods Programs Biomed 113: 210-220.
-
Tarvainen MP, Ranta-Aho PO, Karjalainen PA (2002) An advanced detrending method with application to HRV analysis.IEEE Trans Biomed Eng 49: 172-175.
-
Weber EJ, Molenaar PC, van der Molen MW (1992) Anonstationarity test for the spectral analysis of physiological time series with an application to respiratory sinus arrhythmia.Psychophysiology 29: 55-65.
-
Montano N, Porta A, Cogliati C, Costantino G, Tobaldini E, et al. (2009) Heart rate variability explored in the frequency domain: a tool to investigate the link between heart and behavior.NeurosciBiobehav Rev 33: 71-80.
-
Goldstein DS, Bentho O, Park MY, Sharabi Y (2011) Low-frequency power of heart rate variability is not a measure of cardiac sympathetic tone but may be a measure of modulation of cardiac autonomic outflows by baroreflexes.ExpPhysiol 96: 1255-1261.
-
Balocchi R, Cantini F, Varanini M, Raimondi G, Legramante JM, et al. (2006) Revisiting the potential of time-domain indexes in short-term HRV analysis.Biomed Tech (Berl) 51: 190-193.
-
Sollers JJ 3rd, Buchanan TW, Mowrer SM, Hill LK, Thayer JF (2007) Comparison of the ratio of the standard deviation of the R-R interval and the root mean squared successive differences (SD/rMSSD) to the low frequency-to-high frequency (LF/HF) ratio in a patient population and normal healthy controls. Biomed SciInstrum43: 158-163.
-
Hui-Min Wang and Sheng-Chieh Huang (2012)SDNN/RMSSD as a Surrogate for LF/HF: A Revised Investigation.Modellingand Simulation in Engineering.
-
Fenici R, Brisinda D, Sorbo AR (2011) Methods of real-time assessment of operational stress during realistic police tactical training. In: Kitaeff J (ed). Handbook of Police Psychology. Routledge, New York: 295-319.
-
Berntson GG, Bigger JT Jr, Eckberg DL, Grossman P, Kaufmann PG, et al. (1997) Heart rate variability: origins, methods, and interpretive caveats.Psychophysiology 34: 623-648.
-
Brennan M, Palaniswami M, Kamen P (2002) Poincare plot interpretation using a physiological model of HRV based on a network of oscillators.Am J Physiol Heart CircPhysiol 283: H1873-1886.
-
Chua KC, Chandran V, Acharya UR, Lim CM (2008) Computer-based analysis of cardiac state using entropies, recurrence plots and Poincare geometry.J Med EngTechnol 32: 263-272.
-
Eckmann JP, Oloffson S, Kamphorst SO (1987) Recurrence Plots of Dynamical Systems. EurophysLett 4: 973-977.
-
SPSS Inc. Released 2004. SPSS Statistics for Windows, Version 13.0. Chicago: SPSS Inc.
-
Larsen RJ, Marx ML (2012) An Introduction To Mathematical Statistics and Its Application. Boston, Mass, USA: Pearson Education.
-
McNeil BJ, Keller E, Adelstein SJ (1975) Primer on certain elements of medical decision making.N Engl J Med 293: 211-215.
-
Melillo P, Bracale M, Pecchia L (2011) Nonlinear Heart Rate Variability features for real-life stress detection. Case study: students under stress due to university examination.Biomed Eng Online 10: 96.
-
ALESSI R, NUSYNOWITZ M, ABILDSKOV JA, MOE GK (1958) Nonuniform distribution of vagal effects on the atrial refractory period.Am J Physiol 194: 406-410.
-
Michelucci A, Lazzeri C, Padeletti L, Bagliani G, Colella A, et al. (2001) Altered values of heart rate variability in patients with relapse of atrial fibrillation during the first week after electrical cardioversion: preliminary data.Ital Heart J 2: 435-440.
-
Alcaraz R, Rieta JJ (2008) A non-invasive method to predict electrical cardioversion outcome of persistent atrial fibrillation.Med BiolEngComput 46: 625-635.
-
Alcaraz R, Rieta JJ, Hornero F (2009) Non-invasive atrial fibrillation organization follow-up under successive attempts of electrical cardioversion.Med BiolEngComput 47: 1247-1255.
-
Alcaraz R, Hornero F, Rieta JJ (2011) Noninvasive time and frequency predictors of long-standing atrial fibrillation early recurrence after electrical cardioversion. Pacing ClinElectrophysiol 34: 1241-1250.
-
Janse van Rensburg DC1, Ker JA, Grant CC, Fletcher L (2012) Autonomic impairment in rheumatoid arthritis.Int J Rheum Dis 15: 419-426.
-
da Silva VJ, Gnecchi-Ruscone T, Bellina V, Oliveira M, Maciel L, et al. (2012) Acute adenosine increases cardiac vagal and reduces sympathetic efferent nerve activities in rats.ExpPhysiol 97: 719-729.
-
Hauptman PJ, Schwartz PJ, Gold MR, Borggrefe M, Van Veldhuisen DJ, et al. (2012) Rationale and study design of the increase of vagal tone in heart failure study: INOVATE-HF.Am Heart J 163: 954-962.
-
Zhao M, Sun L, Liu JJ, Wang H, Miao Y, et al. (2012) Vagal nerve modulation: a promising new therapeutic approach for cardiovascular diseases.ClinExpPharmacolPhysiol 39: 701-705.
-
Usui S, Toda N (1991) An overview of biological signal processing: non-linear and non-stationary aspects.Front Med BiolEng 3: 125-129.
-
Beckers F, Verheyden B, Aubert AE (2006) Aging and nonlinear heart rate control in a healthy population.Am J Physiol Heart CircPhysiol 290: H2560-2570.
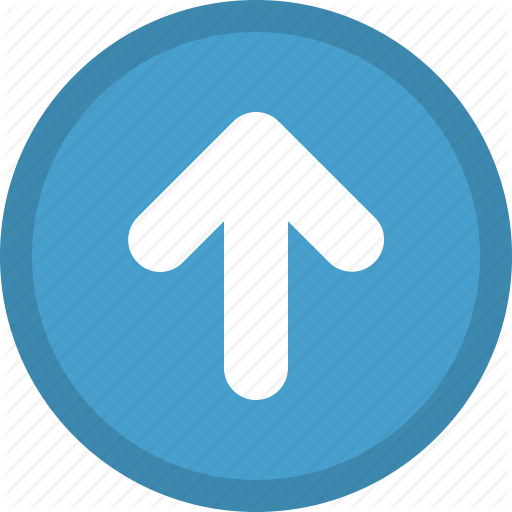