International Journal of Pathology and Clinical Research
BerEP4 and AE1/3 are Reliable Markers of Epithelial Content for Biomarker Discovery Using Reverse Phase Protein Arrays (RPPA)
Ola H Negm1,2, James C Hassall3,4, Wakkas Fadhil3,4, Alain Pitiot4,5, Patrick J Tighe1, Cedric Marchessoux6 and Mohammad Ilyas3,4*
1Immunology, School of Life Sciences, University of Nottingham, Nottingham, UK
2Medical Microbiology and Immunology Department, Faculty of Medicine, Mansoura University, Mansoura, Egypt
3Academic Unit of Molecular Pathology, School of Medicine, University of Nottingham, Nottingham, UK
4Nottingham Molecular Pathology Node, University of Nottingham, Nottingham, UK
5School of Psychology, University of Nottingham, Nottingham, UK
6BARCO NV, President Kennedy Park 35, 8500 Kortrijk, Belgium
*Corresponding author:
Mohammad Ilyas, Academic Unit of Molecular Pathology, The University of Nottingham, Queen's medical centre, Nottingham NG7 2UH, UK, Tel: 0115 82 30735; E-mail: mohammad.ilyas@nottingham.ac.uk
Int J Pathol Clin Res, IJPCR-2-031, (Volume 2, Issue 2), Mini Review; ISSN: 2469-5807
Received: January 08, 2016 | Accepted: March 28, 2016 | Published: April 01, 2016
Citation: Negm OH, Hassall JC, Fadhil W, Pitiot A, Tighe PJ, et al. (2016) BerEP4 and AE1/3 are Reliable Markers of Epithelial Content for Biomarker Discovery Using Reverse Phase Protein Arrays (RPPA). Int J Pathol Clin Res 2:031. 10.23937/2469-5807/1510031
Copyright: © 2016 Negm OH, et al. This is an open-access article distributed under the terms of the Creative Commons Attribution License, which permits unrestricted use, distribution, and reproduction in any medium, provided the original author and source are credited.
Abstract
Background: Reverse phase protein array (RPPA) allows precise protein quantification. Use of homogenised samples confounds interpretation due to uncertainty of cellular content. We hypothesised that antibodies AE1/3 (Type I/II keratins) and BerEP4 (EpCAM) could help to quantify epithelium in tissue from colorectal cancers (CRC).
Methods: AE1/3 and BerEP4 expression was quantified using RPPA and immunohistochemistry (IHC) in formalin-fixed paraffin-embedded tissues from 19 CRCs. For RPPA, samples were tested in sextuplicate. IHC was quantified by transforming images into positive/negative pixels using ImageJ.
Results: In RPPA, the BerEP4 expression was greater than AE1/3 (p < 0.001). There was excellent precision with a mean coefficient of variation of 5.9% for AE1/3 and 6.9% for BerEP4. Expression of AE1/3 and BerEP4 showed significant correlation (p < 0.0001). For IHC, antibodies showed specific epithelial staining. There was no difference in intensity of expression but there were slight differences in extent of expression with, on average, 3.5% more AE1/3-positive pixels than BerEP4-positive pixels (p = 0.016). Antibody expression (number of positive pixels) showed significant correlation (p < 0.0001). Comparison of RPPA with IHC showed significant correlation for each antibody, (BerEP4: p = 0.044; AE1/3: p = 0.037). Combining markers (using the geometric mean) improved the correlation between the assays (p = 0.028).
Conclusion: Expression of AE1/3 and BerEP4 correlate with each other in both RPPA and IHC. For each antibody, the two assays show significant correlation. Either antibody can be used to quantify tumour epithelium in RPPA with improved performance if markers are combined. Normalising RPPA data to "epithelial housekeepers" may improve biomarker data interpretation.
Keywords
Reverse Phase Protein Array (RPPA), Immunohistochemistry (IHC), BerEP4, AE1/3, protein quantification, ImageJ, Colorectal cancer (CRC)
Introduction
Biomarker studies are notorious for their high failure rate. Despite huge investment by both academia and industry, very few biomarkers make the transition from interesting laboratory data to tests with clinical utility. The reasons for this are manifold [1,2]. Some biomarkers are “true” biomarkers inasmuch as they are robust and reproducible but the information they provide does not help to improve patient management beyond that informed by traditional clinical parameters. In such cases, the lack of success of the validated biomarker has to be accepted as part of the vicissitudes of science. In other cases, the biomarker is doomed to failure due to using an inappropriately defined and limited test population, insufficiently powered studies or technical weaknesses (at either the pre-analytical, analytical or post analytical stage).
These issues need to be addressed to increase the chance of successful biomarker discovery. Variability in the analytical stage can be reduced through use of automated assays. This would ensure that each of the technical steps of the assay is identical, the algorithms for quantifying the data are free of observer variability and they can be adapted for high throughput analysis. Reverse Phase Protein Array (RPPA) represents a high-throughput technique that allows simultaneous precise quantification of protein expression in a large number of biological samples [3-5]. Over the past decade, RPPA has been successfully used for different applications including basic and preclinical research, biomarker discovery and clinical trials [6-10].
RPPA is a “morphology-independent” assay i.e. extracts of homogenised tissue are interrogated. Since composition of tissue is variable, the interpretation of the data is confounded by uncertainty regarding the actual cellular contents of the tissue. This can be obviated by histological review of each sample prior to use but creates further conundrums: (i) the speed of acquisition of data is reduced (which may be important when large studies are being undertaken), (ii) there is observer variation in the review and (iii) there may still be uncertainty due to differences between the reviewed section and the actual tissue being tested. Thus a solution is required which would allow capture of all the requisite data in as objective a manner as possible.
In many assays, the problem of variation in the templates is dealt with by a process known as "normalisation" [11-15]. A surrogate "reference" marker which is thought to represent the variable feature is chosen and used as a measure of the feature. For example, in real-time quantitative PCR assays, uncertainty about the amount of nucleic acid template can be reduced by testing for a "housekeeping" gene. This is a gene which is unchanging (in either expression or copy number - depending on the assay) and therefore the "quantity" of gene is linearly related to the amount of template [16].
We hypothesised that the epithelial content of a tissue sample could be quantified with a reference marker for epithelium in an RPPA based experiment.This would allow expression of biomarkers to be normalized to the amount of epithelium present within tissue samples. BerEP4 and AE1/AE3 (AE1/3) are commonly used in routine diagnostic histopathology in a qualitative manner as markers of epithelium. BerEP4 is a transmembrane glycoprotein which detects an epitope on Epithelial Cell Adhesion Molecule (EpCAM, CD326). AE1/3 is a cocktail of two monoclonal antibodies which detect epitopes on Type I and Type II keratins which are present in the cytoskeleton of epithelial cells [17,18]. We reasoned that these would be good antibodies since expression of these markers is maintained in malignancy and, in routine diagnostic practice, they are usually used when tumours are so poorly differentiated that are not obviously epithelial in origin [19].
In this study we investigated whether the quantification of epithelium using BerEP4 and AE1/3 in RPPA reflected that seen by immunohistochemistry (IHC).
Materials and Methods
Tissue samples
Nineteen equential cases of colorectal cancer (CRC) were selected for study. Each case was reviewed by MI and a single tumour blocks was chosen for testing. Formalin-fixed paraffin-embedded (FFPE) tumour tissue was used for both RPPA and IHC. Approval of the study and access to the tissue were granted by Nottingham Health Sciences Biobank.
Protein extraction for RPPA
For protein extraction, 20 μm thick sections were cut from formalin-fixed paraffin-embedded (FFPE) tumour blocks. Tissue was deparaffinised in xylene and the xylene was removed using graded ethanol (100%, 96%, and 70%). Excess alcohol was removed by centrifugation at 16,000g for two minutes. Next, 40 μl of lysis buffers (20% SDS, 0.5 M DTT and 0.5 M Tris-HCl solution at pH 8) were added to the tissue pellet and incubated at 100°C for 60 minutes using a thermomixer. Finally, the tubes were centrifuged at 14,000 rpm for 20 minutes and the supernatants were collected and stored at 80°C until used.
Protein quantification
Protein in the extracted lysates was quantified as described previously [20,21]. In brief, lysates were robotically spotted in triplicate onto nitrocellulose-coated glass slides (Grace Bio-labs, USA) with a micro arraying robot (MicroGrid 610, Digilab, Marlborough, MA, USA). In addition, serial two-fold dilutions of 1.0 mg/mL bovine serum albumin (Sigma Aldrich, UK) were spotted onto the array as a total protein standard. Printed arrays were stained with Fast Green Stain (0.005% FCF (Sigma-Aldrich, UK) in 30% ethanol, 10% acetic acid and 60% water, V/V/V) for 45 min at room temperature on a rocking platform at 40 rpm. Destaining was performed 2X 15 min each with 30% ethanol, 10% acetic acid and 60% water. After washing, the slide was air dried and scanned at 700 nm using an Odyssey scanner (LI-COR, Lincoln, USA).
Reverse Phase Protein Microarray (RPPA)
RPPA methodology has been described previously [22]. In brief, equal amounts of protein lysates (1 μg/μl) were loaded into a 384-well plate. Samples were robotically spotted in sextuplicate onto nitrocellulose-coated glass slides. Blocking of slides was performed by incubating the arrays overnight at 4°C with Super G blocking buffer (Grace Bio-labs, USA). Next, the arrays were incubated with the primary antibodies (Table 1) overnight at 4°C with agitation at 20 rpm. After three washes of 5 min each with Tris Buffer Saline (TBS) / 0.5% Tween 20, slides were incubated with infrared Licor secondary antibodies (680 CW anti-mouse Ig antibody, Cat number: 926-65010) diluted 1:5000 in washing buffer for 30 minutes at room temperature in the dark. Arrays were visualized using an Odyssey high-resolution scanner (LI-COR, Lincoln, USA) at 21 μm resolution using the 700 nm (red) channel. The Axon Genepix Pro-6 Microarray Image Analysis software was used to determine the average fluorescence intensities of all spots on each array. Protein intensity signals were finally determined with background subtraction using RPP analyzer, a module within the R statistical language on the CRAN (http://cran.r-project.org) [23]. For comparative analysis, BerEP4 and AE1/3 intensity signals were normalised to the protein concentration of the lysate.
Table 1: List of antibodies used in RPPA and IHC.
View Table 1
Immunohistochemistry (IHC)
Whole tissue sections from each tumour block were cut in duplicate and fixed to specially coated slides by baking for 20 minutes at 60°C. Antibody information for BerEP4 and for AE1/3 is located in Table 1. IHC was performed using a BenchMark ULTRA automated IHC/ISH slide staining system (Ventana, Roche) in accordance with the manufacturer's instructions. All materials came with the UltraView(UV) immunostaining kit (Ventana, Roche). The following specific incubation steps were used for the procedure:antigen retrieval for 8 minutes; UV inhibitor for 4 minutes; primary antibody for 32 minutes (see dilutions in Table 1); UV Horse-Radish Peroxidase UNIV MULT for 8 minutes; UV 3'3' Diaminobenzidine (DAB) and UV DAB H2O2 for 8 minutes; UV Copper for 4 minutes; Haematoxylin II for 12 minutes.
Image Analysis
Digital images of the immunostained slides were obtained using the Nanozoomer (Hamamatsu). Slides were scanned in at X40 and saved in the proprietary .ndpi format. The images were viewed using the NDPIv2 viewing software (Hamamatsu) and exported for image analysis in JPEG format at magnification X0.3. Images were analysed using the publicly available software ImageJ (http://imagej.nih.gov/ij/) and two features were evaluated i.e. mean intensity of pixels and number of positively stained pixels. Images were opened in ImageJ and were processed using the colour deconvolution plugin. This plugin is able to extract colours and specifically separates the image into 3 images i.e. "colour 1" an image containing the haematoxylin colour, "colour 2" an image containing the DAB brown colour and "colour 3" containing the remaining colours (Figure 1). Analysis was restricted to "colour 2" and a histogram was obtained of each image showing the mean pixel intensity. In order to quantify the number of positively staining pixels, the image was processed using the "Make Binary" function in ImageJ to create an image which consisted of only black and white pixels (intensity 0 and 255 respectively). The total number of black pixels (corresponding to stained pixels) could then be calculated via the histogram function. For comparative analysis it is necessary to normalise the number of positive pixels to the total number of pixels comprising the tissue section (indicating the proportion of tissue containing tumour). For this, the polygon selection tool was used to outline the tissue area and total number of pixels in this area were ascertained via the histogram function.
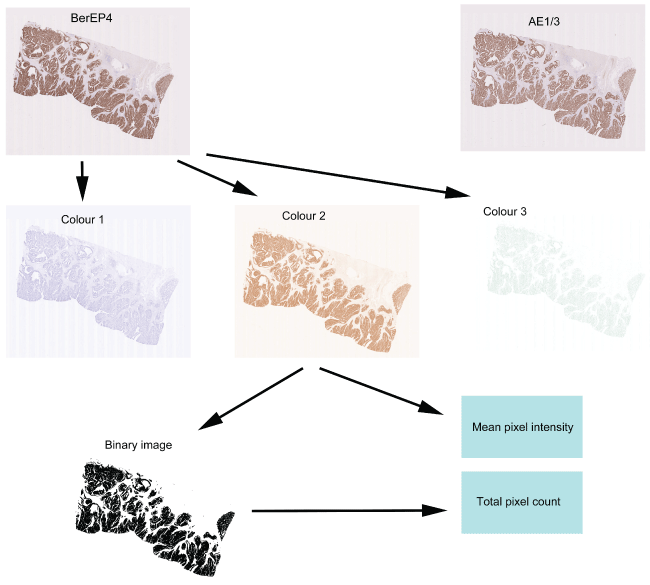
.
Figure 1: Quantification of the immunohistochemistry. Two sequential sections stained with BerEP4 and AE1/3 are shown at the top and show near identical patterns of expression. The process of quantification is shown for the section stained with BerEP4. The section initially undergoes colour deconvolution to extract the colours of Haematoxylin (Colour 1), DAB (Colour 2) and the remainder (Colour 3). From Colour 2, the mean pixel intensity can be calculated. The Colour 2 image then undergoes binary transformation and all the brown-stained pixels are converted to black and all the non-stained pixels are converted to white. The number of black pixels then indicates the area showing positive staining.
View Figure 1
Statistical analysis
Statistical analysis was undertaken via the use of GraphPad Prism 6.5. Precision of the RPPA assay was tested using raw data to calculate the coefficient of variation (CV). For intensity of IHC, the mean value of pixel intensity for "Colour 2" was used. For all other tests of the RPPA and IHC, raw data were firstly normalised (see above) and normalised values were then transformed using the square-root [24] method to allow use of parametric tests. Normal distribution was confirmed by the Shapiro-Wilk test and comparative analysis was performed using paired t-test and linear regression analysis. In order to assess correlation of RPPA and IHC using both markers in combination, the geometric mean (calculated by the square root of the product of the values for each biomarker [25]) was used. A value of p < 0.05 was considered significant.
Results
Precision of BerEP4 and AE1/3 in the RPPA assay
The use of BerEP4 and AE1/3 as robust and reliable markers of epithelium in routine diagnostic practice is well established. In order to evaluate their utility in RPPA, we firstly tested the precision (i.e. reproducibility of data in simultaneous replicates) of these antibodies. Each of the 19 cases of CRC was spotted as 6 replicates onto the array and BerEP4 had a mean coefficient of variation (CV) of 6.9% whilst AE1/3 had a CV of 5.9%. This degree of precision is excellent for this kind of assay and indicates that these antibodies perform robustly in RPPA [26].
Quantification of epithelium by RPPA
The normalised and transformed RPPA data for BerEP4 and AE1/3 are shown in Table 2. Comparison of the normalised fluorescence data showed that the intensity of fluorescence was greater for BerEP4 than AE1/3 with a mean fold difference of 17. Pairwise comparison of the samples (using a paired T-test) showed that this difference was statistically significant. The expression of the two antibodies was significantly correlated (Figure 2, Pearson's correlation coefficient r = 0.9752, p < 0.0001, 95% CI = 0.9351 to 0.9906).
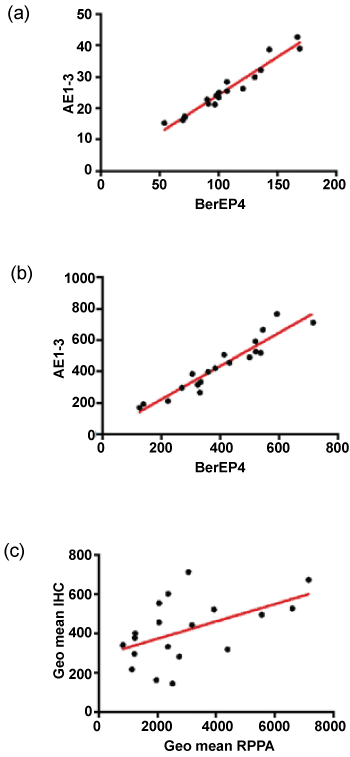
.
Figure 2: Comparison of antibodies and assays. (a&b) show correlation of AE1/3 and BerEP4 in RPPA and IHC respectively; (c) shows the inter-assay correlation when the geometric means are plotted. There is a significant correlation for each of the antibodies individually (not shown) but the performance is better when the markers are combined.
View Figure 2
Table 2: Biomarker data.
View Table 2
Quantification of epithelium by IHC and comparison with RPPA
The IHC staining for both antibodies was reviewed by MI and showed strong and specific staining in the epithelium with little staining in the stroma (Figure 1). The digital data were confirmed as normal and pairwise comparison of mean pixel intensity values showed no difference in intensity of staining between the two antibodies. The number of stained pixels represents the area stained by the antibodies and comparison of the number of stained pixels (normalised to the total number of pixels in the tissue section) showed that, on average, there were 3.5% more AE1/3-positive pixels than BerEP4-positive pixels (p = 0.016, paired t-test on transformed data). As with the RPPA, the expression of the two antibodies was significantly correlated (Figure 2, Pearson's correlation coefficient r = 0.9484, p < 0.0001, 95% CI = 0.8681 to 0.9803). Although RPPA and IHC represent two completely different methodologies, it was of importance to see if the data between the two showed correlation. For each of the antibodies, there was statistically significant correlation (for BerEP4: Pearson's correlation coefficient r = 0.4652, 95%CI 0.01-0.76, p = 0.044); for AE1/3, Pearson's correlation coefficient r = 0.48, 95%CI 0.03 to 0.77, p = 0.037). As we had used 2 different markers, we sought to combine data. The geometric mean of BerEP4 and AE1/3 for RPPA and IHC were plotted and showed a significant correlation (Figure 2, Pearson's correlation coefficient r = 0.50, 95%CI = 0.06095 to 0.7783, p= 0.028). The correlation of the geometric means was confirmed by randomly resampling the data (Supplementary Figure).
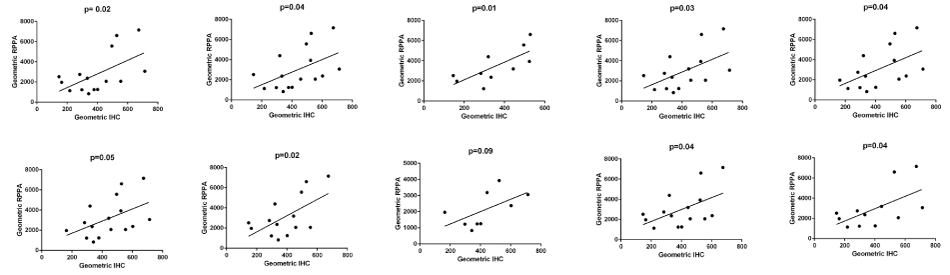
.
Supplementary Figure: In order to further validate the data, 10 random sub-samples were taken from the data set and correlation between RPPA and IHC was tested (Bischl, B. et al., Resampling Methods for Meta-Model Validation with Recommendations for Evolutionary Computation. Evolutionary Computation, 2012. 20(2): p. 249-275). In 9/10 data sub-sets, there was a significant correlation. The one time it was not significant was when the data set was very small.
View Supplementary Figure
Discussion
RPPA is a powerful method of quantifying proteins but it is morphology-independent. Data are therefore confounded by uncertainty around the actual cellular composition of the tissue sample. An epithelial housekeeper or reference marker accurately reflecting epithelial contents of a tissue sample would allow biomarker expression to be normalised to epithelial contents and could improve data analysis. BerEP4 and AE1/3 are well established in routine diagnostic practice as robust qualitative markers of epithelium and this was indeed confirmed by our immunostaining in this study. However, it is well known that performance of antibodies in different assays may vary markedly - thus an antibody which performs well in immunohistochemistry may not perform well in Western blot [27]. In order to assess the utility of BerEP4 and AE1/3 as quantitative markers in RPPA assays, we firstly evaluated the precision (i.e. reproducibility) of these antibodies. Our data showed that the mean coefficient of variation (CV) for AE1/3 was 5.9% and BerEP4 was 6.9%. The CV is frequently used as an indicator of the precision of an assay and a value of less than 10% is regarded as acceptable [26]. The data therefore indicate that the antibodies perform consistently in RPPA. For every sample, the level of expression of BerEP4 in RPPA was higher than that of AE1/3 (p < 0.001) with a mean 17-fold difference. This would suggest that the protein detected by BerEP4 is present in greater amounts than that detected by AE1/3 although it is possible that it represents differences in antibody properties (e.g. affinity or avidity for the antigen). In the IHC, the results were reversed. There was no difference in the mean intensity of staining between the 2 groups but the epithelial content quantified by AE1/3 was, on average, 3.5% greater than that quantified by BerEP4 (p = 0.016). The difference between the 2 antibodies in the IHC is relatively trivial but it does confirm other data suggesting the poorly quantitative nature of the IHC assay [28,29]. There was a significant correlation between the two antibodies in both RPPA and IHC. Even though they are measuring different proteins, this implies that the proteins are common to a particular component in the tissue. The IHC demonstrates that the component is the epithelium and it can thus be inferred it is the same in the RPPA assay. As well as being precise, the RPPA assay using BerEP4 and AE1/3 needs to be accurate. We therefore validated the RPPA against the IHC. The intensity of fluorescence for both antibodies in the RPPA was significantly correlated with positive pixel count in the IHC (BerEP4, p = 0.044, AE1/3 p = 0.037). Combining the biomarkers improved the correlation between the two assays (p = 0.028). This confirms that these antibodies can be used to quantify relative epithelial content in homogenised tissues lysates. This, in turn, means that these antibodies can be used to "normalise" biomarker expression in RPPA thus, to some degree, reducing the uncertainty surrounding cellular content in tissue samples.
Our data show that, where expression of a biomarker is known to be restricted to the epithelium, performing an assay with these antibodies will obviate the need for morphological assessment of tissue. However, there are two caveats: (i) where it is possible that the biomarker may be expressed in other tissue compartment, optimal data analysis will continue to be morphology-dependent i.e. tissue samples will need to be reviewed histologically in order to ascertain which tissue compartment is expressing the biomarker of interest and at what level and (ii) these two antibodies are excellent epithelial markers in colorectal tissue. Tumours arising in other organs may require different epithelial housekeepers.
In summary, our data suggest BerEP4 and AE1/3 can be used for the relative quantification of epithelium in RPPA. By inference, other tissue compartments could similarly be evaluated. This allows expression of biomarkers tested using RPPA to be normalised to epithelial content possibly improving analysis and increasing the chance of biomarker discovery.
Acknowledgement
We would like to thank Claire Hawkes for supporting the immunohistochemistry. This work was supported by the University of Nottingham and the AIDPATH consortium (http://aidpath.eu/; FP7 programme under grant agreement No 612471).
References
-
Diamandis EP (2012) The failure of protein cancer biomarkers to reach the clinic: why, and what can be done to address the problem? BMC Med 10: 87.
-
Kern SE (2012) Why your new cancer biomarker may never work: recurrent patterns and remarkable diversity in biomarker failures. Cancer Res 72: 6097-6101.
-
Berg D, Wolff C, Malinowsky K, Tran K, Walch A, et al. (2012) Profiling signalling pathways in formalin-fixed and paraffin-embedded breast cancer tissues reveals cross-talk between EGFR, HER, HER3 and uPAR. J Cell Physiol 227: 204-212.
-
Gallagher RI, Espina V (2014) Reverse phase protein arrays: mapping the path towards personalized medicine. Mol Diagn Ther 18: 619-630.
-
Masuda M, Yamada T (2015) Signaling pathway profiling by reverse-phase protein array for personalized cancer medicine. Biochim Biophys Acta 1854: 651-657.
-
Aleskandarany MA, Negm OH, Rakha EA, Ahmed MA, Nolan CC, et al. (2012) TOMM34 expression in early invasive breast cancer: a biomarker associated with poor outcome. Breast Cancer Res Treat 136: 419-427.
-
Cheng H, Fertig EJ, Ozawa H, Hatakeyama H, Howard JD, et al. (2015) Decreased SMAD4 expression is associated with induction of epithelial-to-mesenchymal transition and cetuximab resistance in head and neck squamous cell carcinoma. Cancer Biol Ther 16: 1252-1258.
-
Park ES, Rabinovsky R, Carey M, Hennessy BT, Agarwal R, et al. (2010) Integrative analysis of proteomic signatures, mutations, and drug responsiveness in the NCI 60 cancer cell line set. Mol Cancer Ther 9: 257-267.
-
Sonnenblick A, Brohée S, Fumagalli D, Vincent D, Venet D, et al. (2015) Constitutive phosphorylated STAT3-associated gene signature is predictive for trastuzumab resistance in primary HER2-positive breast cancer. BMC Med 13: 177.
-
Sonntag J, Schlüter K, Bernhardt S, Korf U (2014) Subtyping of breast cancer using reverse phase protein arrays. Expert Rev Proteomics 11: 757-770.
-
Bolstad BM, Irizarry RA, Astrand M, Speed TP (2003) A comparison of normalization methods for high density oligonucleotide array data based on variance and bias. Bioinformatics 19: 185-193.
-
Engelen K, Coessens B, Marchal K, De Moor B (2003) MARAN: normalizing micro-array data. Bioinformatics 19: 893-894.
-
Grewal A, Lambert P, Stockton J (2007) Analysis of expression data: an overview. Curr Protoc Bioinformatics Chapter 7: Unit 7.
-
Quackenbush J (2002) Microarray data normalization and transformation. Nat Genet 32 Suppl: 496-501.
-
Wu W, Dave N, Tseng GC, Richards T, Xing EP, et al. (2005) Comparison of normalization methods for CodeLink Bioarray data. BMC Bioinformatics 6: 309.
-
Dydensborg AB, Herring E, Auclair J, Tremblay E, Beaulieu JF, et al. (2006) Normalizing genes for quantitative RT-PCR in differentiating human intestinal epithelial cells and adenocarcinomas of the colon. Am J Physiol Gastrointest Liver Physiol 290: G1067-74.
-
Sheibani K, Shin SS, Kezirian J, Weiss LM (1991) Ber-EP4 antibody as a discriminant in the differential diagnosis of malignant mesothelioma versus adenocarcinoma. Am J Surg Pathol 15: 779-784.
-
Tseng SC, Jarvinen MJ, Nelson WG, Huang JW, Woodcock-Mitchell J, et al. (1982) Correlation of specific keratins with different types of epithelial differentiation: monoclonal antibody studies. Cell 30: 361-72.
-
Goddard MJ, Wilson B, Grant JW (1991) Comparison of commercially available cytokeratin antibodies in normal and neoplastic adult epithelial and non-epithelial tissues. J Clin Pathol 44: 660-663.
-
Loebke C, Sueltmann H, Schmidt C, Henjes F, Wiemann S, et al. (2007) Infrared-based protein detection arrays for quantitative proteomics. Proteomics 7: 558-564.
-
Mueller C, Edmiston KH, Carpenter C, Gaffney E, Ryan C, et al. (2011) One-step preservation of phosphoproteins and tissue morphology at room temperature for diagnostic and research specimens. PLoS One 6: e23780.
-
Negm OH, Mannsperger HA, McDermott EM, Drewe E, Powell RJ, et al. (2014) A pro-inflammatory signalome is constitutively activated by C33Y mutant TNF receptor 1 in TNF receptor-associated periodic syndrome (TRAPS). Eur J Immunol 44: 2096-2110.
-
Mannsperger HA, Gade S, Henjes F, Beissbarth T, Korf U (2010) RPPanalyzer: Analysis of reverse-phase protein array data. Bioinformatics 26: 2202-2203.
-
Bartlett MS (1936) The Square Root Transformation in Analysis of Variance. Supplement to the Journal of the Royal Statistical Society 3: 68-78.
-
Vandesompele J, De Preter K, Pattyn F, Poppe B, Van Roy N, et al. (2002) Accurate normalization of real-time quantitative RT-PCR data by geometric averaging of multiple internal control genes. Genome Biol 3: RESEARCH0034.
-
Maecker HT, Hassler J, Payne JK, Summers A, Comatas K, et al. (2008) Precision and linearity targets for validation of an IFNgamma ELISPOT, cytokine flow cytometry, and tetramer assay using CMV peptides. BMC Immunol 9: 9.
-
Marx V (2013) Finding the right antibody for the job. Nat Meth 10: 703-707.
-
Levenson RM, Borowsky AD, Angelo M2 (2015) Immunohistochemistry and mass spectrometry for highly multiplexed cellular molecular imaging. Lab Invest 95: 397-405.
-
Rimm DL (2006) What brown cannot do for you. Nat Biotechnol 24: 914-916.
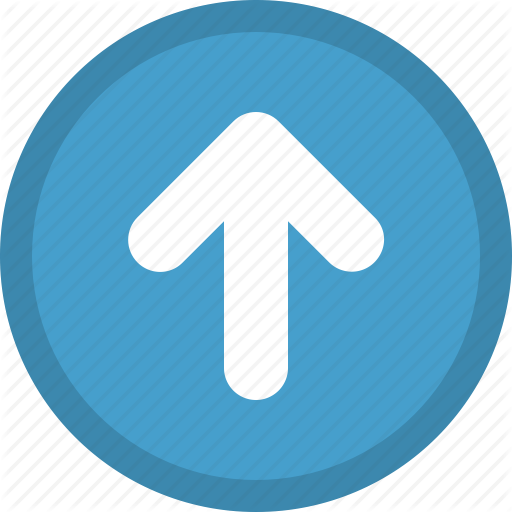