International Journal of Diabetes and Clinical Research
Hba1c Variability, Absolute Changes in Hba1c, and the Risk of Poor Glycemic Control among Older Adults with Diabetes Enrolled in Medicare Advantage Plans
Dingwei Dai1*, Susannah Higgins1 and Natan Szapiro2
1Clinical Analytics, Informatics Department, Independence Blue Cross, Philadelphia, PA, USA
2Quality and Risk Management, Informatics Department, Independence Blue Cross, Philadelphia, PA, USA
*Corresponding author:
Dingwei Dai, PhD, Lead Research Scientist, Department of Informatics, Independence Blue Cross, 1901 Market Street, Philadelphia, PA19103, USA, Tel: 215-640-7480, E-mail: Dingwei.dai@ibx.com
Int J Diabetes Clin Res, IJDCR-3-063, (Volume 3, Issue 3), Research Article; ISSN: 2377-3634
Received: April 14, 2016 | Accepted: June 22, 2016 | Published: July 01, 2016
Citation: Dai D, Higgins S, Szapiro N (2016) Hba1c Variability, Absolute Changes in Hba1c, and the Risk of Poor Glycemic Control among Older Adults with Diabetes Enrolled in Medicare Advantage Plans. Int J Diabetes Clin Res 3:063. 10.23937/2377-3634/1410063
Copyright: © 2016 Dai D, et al. This is an open-access article distributed under the terms of the Creative Commons Attribution License, which permits unrestricted use, distribution, and reproduction in any medium, provided the original author and source are credited.
Abstract
Objective: To evaluate association of visit-to-visit HbA1c variability and absolute changes in HbA1c with poor glycemic control in patients with diabetes.
Study design: A retrospective cohort study.
Methods: Healthcare claims data for all diabetes patients aged 65 years or older and continuously enrolled in Medicare Advantage plans in Independence Blue Cross from 2013 to 2014 and had ≥ 3 HbA1c measurements in the 2013 measurement year (MY). We used patients' zip codes to link to US census data to get socioeconomic status information. Poor glycemic control was identified as the most recent HbA1c level > 9.0% during the MY. HbA1c variability was defined as within-subject standard deviation of HbA1c levels; absolute change in HbA1c was defined as the difference between the most recent HbA1c level and the first HbA1c level measured in MY2013. Multivariable logistic regression models were created, with poor glycemic control in MY2014 as the outcome.
Results: Among the cohort composed of 4,458 patients enrolled in the plans in both 2013 and 2014, the mean age was 78.3 years, with 45.6% male. A total of 21.9% of patients had poor glycemic control in MY2014. Increasing quintiles of HbA1c variability are associated with both increased mean HbA1c and absolute change in HbA1c (both p < 0.0001). The proportion of poor glycemic control increased with increased quintile of HbA1c variability ( p < 0.0001). After adjusting by confounders, HbA1c variability was not associated with increased poor glycemic control in the subsequent year (adjusted OR = 0.98, 95% CI: 0.69-1.38 for the highest quintile), whereas mean HbA1c and absolute change in HbA1c were both associated (adjusted OR = 1.68 (95% CI: 1.51-1.88) for mean; adjusted OR = 1.14 (95% CI: 1.03-1.26) for change).
Conclusion: Mean HbA1c and absolute change in HbA1c were both associated with poor glycemic control, whereas HbA1c variability was not.
Keywords
HbA1c variability, Glycemic control, Poorly controlled, Risk factors, Diabetes care
Introduction
Diabetes is one of the most common chronic diseases as well as a leading cause of death and disability worldwide [1,2]. Since the Diabetes Control and Complication Trial and the United Kingdom Prospective Diabetes Study demonstrated that excellent glycemic control reduces microvascular complications in types 1 and 2 diabetes mellitus, respectively [3,4]. Glycated hemoglobin A1c (HbA1c) level has become an increasingly important measure of glycemic control. Glycemic controlis one of the important strategies for the management of diabetes to prevent complications and to reduce the overall disease care cost as regarded by the American Diabetes Association [5]. Despite the strong consensus that excellent glycemic control improves diabetes outcomes and reduces health care costs [6], there are a lot of patients with diabetes who still have poor glycemic control [7,8]. The Center for Medicare and Medicaid Services (CMS) uses its Medicare STAR program to monitor and reward the quality of care in health plans with Medicare Advantage enrollees, and provides substantial incentives to health plans that perform well on its Medicare STAR metrics [9]. One among the nine triple-weighted metrics is Diabetes Care-Blood Sugar Controlled (i.e., plan members with diabetes whose blood sugar is under control) [10]. The implementation of the measure emphasizes the responsibility of health plans to monitor and improve glycemic control.
There is emerging interest in examining the influence of HbA1c variability in chronic complications of diabetes [11,12]. Recent studies have found the development of microalbuminuria, progression of nephropathy, and incidence of cardiovascular disease related to HbA1c variability independent of the mean HbA1c level [12-14]. In people with type 2 diabetes, high levels of HbA1c, HbA1c variability, absolute changes in HbA1c might be associated with increased mortality [14-16]. However, HbA1c is not an independent predictor of antidiabetic medication nonadherence [17]. As far as we know, no published population-base studies on relationship of HbA1c variability and poor glycemic control. Therefore, we have investigated poor glycemic control and its relation to both HbA1c variability and absolute changes in HbA1c levels in older adults with diabetes enrolled in Medicare Advantage plans. We therefore aim to refine the ability to identify the patients who are at risk for poor glycemic control in the future then target this population.
Methods
Data source
The Independence Blue Cross (IBC) Active Enterprise Data Warehouse (AEDW) was utilized, consisting of an integrated dataset containing patient demographics, diagnosis, procedures, and detailed pharmacy information as well as laboratory data, including International Classification of Diseases, 9th Revision, Clinical Modification (ICD-9-CM) codes; Current Procedural Terminology (CPT) and Healthcare Common Procedure Coding System (HCPCS) codes for each procedure, National Drug Codes (NDC) for drug dispensed, and Health Care Financing Administration (HCFA) codes for specialty. IBC serves nearly 10 million people in 27 states and the District of Columbia across the United States, including 2.5 million members in southeastern pennsylvania. This retrospective claims data analysis was conducted in compliance with state and federal laws, including the Health Insurance Portability and Accountability Act of 1996. All claims data were from a limited dataset with de-identified patient information. No patients were directly involved in the study; therefore, review by an institutional review board was unnecessary.
Inclusion/Exclusion criteria
To define diabetes, we applied the Healthcare Effectiveness Data and Information Set (HEDIS) codes defined by the National Committee for Quality Assurance (NCQA) [18]. HEDIS defined diabetic patients in the Medicare Advantage plans as members with diabetes (type 1 or type 2) and no more than 1 gap in enrollment of up to 45 days during the MY. To determine continuous enrollment for a Medicare beneficiary for whom enrollment is verified monthly, the member may not have more than a 1-month gap in coverage (i.e., a member whose coverage lapsesfor 2 months [60 days] is not considered as continuously enrolled). Two methods identify members with diabetes: pharmacy data and claims/encounter data. The organization must use both to identify the eligible population, but a member only needs to be identified in 1 to be included in the measure. Members may be identified as having diabetes during the MY or the year prior to the MY [19]. Our study sample consisted of patients with diabetes continuously enrolled in Medicare Advantage plans in IBC from 2013 to 2014 and had ≥ 3 HbA1c measurements in MY2013. We included patient aged 65 years or older. We excluded patients who did not have a diagnosis of diabetes and had a diagnosis of polycystic ovaries, gestational diabetes or steroid-induced diabetes [10].
Data management
Poor glycemic control was identified as the most recent HbA1c level > 9.0% during the MY, per the CMS/NCQA definition [10,18]. HbA1c was defined as within-subject standard deviation of HbA1c levels; absolute change in HbA1c was defined as the difference between the most recent HbA1c and the first HbA1c level measured in MY2013. We used patients' zip codes to link to 2010 US census to get socioeconomic status information. General health status was assessed through the Charlson comorbidity index [20]. The comorbidity index was assessed from claims data for services provided between January 1, 2013 and December 31, 2013; and each claim was billed at least one primary ICD-9 diagnosis code and up to 15 secondary diagnosis codes. The comorbidity index was assessed using the Deyo strategy [21]. The comorbidity index was modified and computed for all diagnoses recorded in all of the claims during the year excluding diabetes because all study subjects had this diagnosis. Comorbidities and complications were identified by ICD-9 diagnosis codes (primary or secondary) and included the following: diabetic retinopathy (362.01; 362.02; 362.10; 379.23; 250.5x), diabetic neuropathy (357.2 or 250.6x), diabetic nephropathy (250.4x), ischemic heart disease (410.xx-413.xx), stroke (430.xx-434.xx and 436.xx), hyperlipidemia (272.0-272.4), chronic kidney disease (CKD) (585.xx), hypertension (401.xx-405.xx), obesity 278.0x), tobacco use (305.1 or V15.82), anxiety (300.xx except 300.3 and 300.4), and depression (311; 296.2; 296.3; 296.5-296.7; 300.4 or 296.82; 296.89). DxCG risk score refers to the concurrent medical risk score calculated using the medical risk adjustment model developed by Verisk Health DxCG Risk solutions, version 3.1 (Verisk Health, Cary, NC) [22]. These models use linear, additive formulas obtained from ordinary least squares regressions to generate predictions from expenses associated with clinical data and demographic factors. The risk score employed here used patient age, gender, and claims information from all medical encounter and enrollment data to estimate annual total medical resource use [22]. We categorized the score as 1 (1st tertile, low risk), 2 (2nd tertile, middle), and 3 (3rd tertile, high risk) in this study. The adherence to diabetic medication was defined by the medication possession ratio (MPR). The MPR is calculated as the sum of total days' supply of the medication from the first to the last prescription fill, divided by the total number of days in this period [23]. The MPR was calculated separately for each drug class for all diabetic medications prescribed for a subject using prescription medication claims data from AEDW. Then, an average MPR was calculated with equal weighting of each drug class. The average antidiabetic medication MPR was dichotomized as non adherent and adherent according to the traditional standard of < 80% and ≥ 80%, respectively [24].
Statistical analysis
We first described the social demographic and clinical characteristics of the patients in this cohort by calculating percentages for categorical variables and media plus interquartile range for continuous variables. Patients were divided into five groups according to the HbA1c variability quintile. Comparisons of social demographic and clinical characteristics of each group were performed using the chi-square test for categorical variables and the one-way ANOVA test for continuous variables. Bivariate associations between covariates and poor glycemic control were tested using the chi-square test for categorical variables and the Wilcoxon rank-sum test for continuous variables. To assess the relationships between HbA1c variability or absolute change in HbA1c and poor glycemic control, we fitted univariate and multivariable logistic regression models. The multivariable regression model included covariates that were statistically significantly associated with poor glycemic controlbased on bivariate associations and variables deemed important to predicting poor glycemic control according to literature review. These variables contained age in years, gender, race, mean A1c, obesity, depression, chronic kidney disease, charlson comorbidity index, DxCG risk score, emergency department visit, number of outpatient visit, any hospitalization, number of PCP/internist visit, number of specialist visit, number of distinct generic drug use, and insulin use during MY2013. For all logistic regression models, the odds ratio (OR) and 95% confidence intervals (CIs) were calculated, and p < 0.05 (two tailed) was used for all significance levels. The Hosmer-Lemeshow test for goodness-of-fit was used to evaluate the models. All data management and statistical analyses were conducted using SAS 9.4 (SAS Institute Inc., Cary, NC).
Results
There were a total of 4,458 diabetes patients aged 65 years or older and continuously enrolled in Medicare Advantage plans in IBC from 2013 to 2014 with at least 3 HbA1c measurements in MY2013 (Table 1). The majority of patients were female (54.4%), the mean age was 78.3 years, 15% of patients with obesity, 86% with hyperlipidemia, 90% with hypertension, 15% with stroke, near 7% with anxiety, 8% with depression, 22% with chronic kidney disease. More than 30% of the patients had at least one emergency room visit. The average number of outpatient visits per patient was two, primary care or intern physician visit was six, and specialist visit was 4. The average number of distinct generic drugs used per patient was ten. More than 70% of the patients were adherent to antidiabetic medications, and 22.7% used insulin. The average within-subject mean HbA1c level was 7% ± 1.1%. Figure 1 shows the individual HbA1c level profile during the MY by HbA1c variability quintile. The group of highest HbA1c variability quintile had the biggest variation across HbA1c measurements within subjects, while the group of lowest HbA1c variability quintile had the smallest variation over HbA1c measurements within subjects. Figure 2 demonstrates the changes in the HbA1c level in the five groups with different HbA1c variability over the MY, illustrating relative stable in average HbA1c lever across measurements in each group. The group of highest HbA1c variability quintile had the highest HbA1c level, while the group of lowest HbA1c variability quintile had the lowest HbA1c level over measurements. Table 1 also shows that increasing quintiles of HbA1c variability are associated with increased mean HbA1c (p < 0.0001).
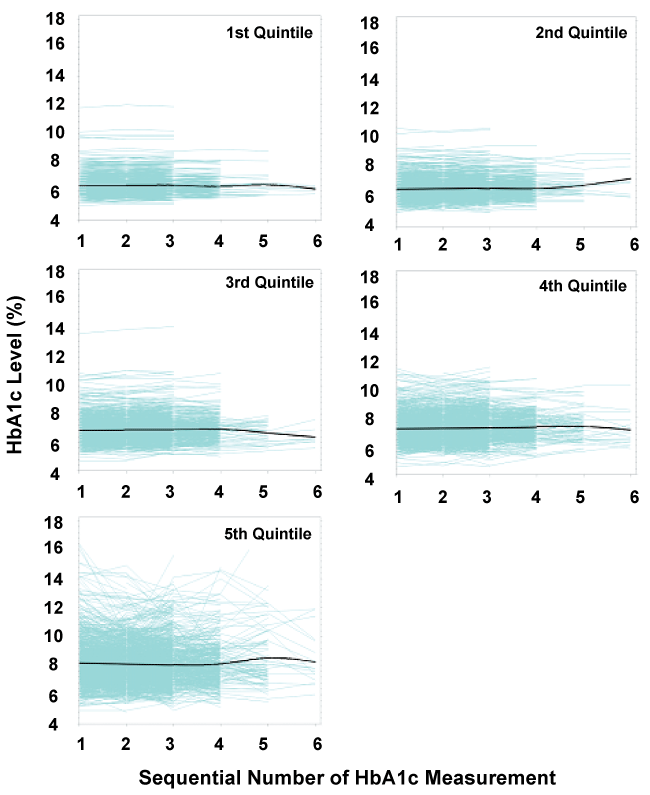
.
Figure 1: Individual HbA1c level profile during the measurement year by HbA1c variability quintile group. Shown are the HbA1c values for each HbA1c measurement, for first measurement up to 6 measurements. The plotted short dash blue lines display the individual HbA1c profiles, and the plotted solid black lines display the average trend in the HbA1c variability quintile group.
View Figure 1
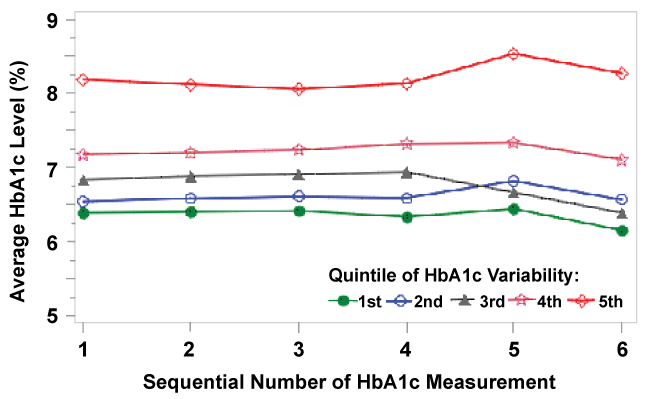
.
Figure 2: Average HbA1c levels by sequential HbA1c measurements according to HbA1c Variability quintile.
View Figure 2
Table 1 shows the social demographic and clinical characteristics of the five groups with different HbA1c variabilities. There were no differences in race, median household income, and education level, plan type (HMO [health maintenance organization] or PPO [preferred provider organization]), or adherent to antidiabetic medications among the groups. However, as the HbA1c variability increased, mean age decreased significantly. The rates of obesity, tobacco use, ischemic heart disease, depression, chronic kidney disease, diabetic complications, modified charlson comorbidity index, DxCG risk score, insulin use, number of distinct generic drug used, and other medical services utilization increased significantly with the increasing quintile of HbA1c variability. As the HbA1c variability increased, the number of HbA1c measurements, mean HbA1c, absolute changes in HbA1c, and the rate of poor glycemic control increase significantly. The HbA1c variability was also closely related to the mean HbA1c of all patients (r = 0.576, p < 0.0001).
Table 1: Association of HbA1c variability and social demographic and clinical characteristics of patients with diabetes.
View Table 1
A total of 21.9% (95% CI: 20.67-23.11) of patients had poor glycemic control in MY2014 in this study cohort. Obesity, depression, chronic kidney disease, insulin use, emergency department visits, higher number of outpatient visits, any hospitalization, higher within-subject mean and within-subject absolute change HbA1c were significantly associated with poor glycemic control (Table 2). No significant associations were found for all other factors such as age, gender, race, household income, education level, tobacco use, hypertension, ischemic heart disease, anxiety, diabetic complications, Charlson comorbidity index and DxCG risk score.
Table 2: Association of poor glycemic control and social demographic and clinical characteristics of member with diabetes.
View Table 2
The proportion of patients who had poor glycemic control increased overall with increasing quintiles of HbA1c variability (15.4%, 15.9%, 18.1%, 22.1%, 28.5%, p < 0.0001 for association; Table 2) . In the unadjusted logistic regression model, there was a moderately strong association between HbA1c variability and poor glycemic control that was statistically significant for the highest quintile (OR = 2.52, 95% CI = 1.91-3.34; Table 3). However, the fully adjusted model showed no association between HbA1c variability and poor glycemic control. Poor glycemic control was significantly associated with mean HbA1c level (OR = 1.70, 95% CI = 1.52-1.90), absolute change in HbA1c level (OR = 1.14, 95% CI = 1.03-1.26), chronic kidney disease (OR = 1.42, 95% CI = 1.08-1.86), number of primary care visits (OR = 0.98, 95% CI = 0.97-0.99), number of specialist visits (OR = 1.02, 95% CI = 1.01-1.04), and insulin use (OR = 1.36, 95% CI = 1.05-1.78). All other independent variables (age, gender, obesity, depression, Charlson comorbidity index, DxCG risk score, emergency department visits, number of outpatient visits, hospitalization, and number of distinct generic drug used) were not significantly associated with poor glycemic control in this study population (all p > 0.05, Table 3).
Table 3: Logistic regression modeling to predict odds of poor glycemic control.
View Table 3
Discussion
We found that HbA1c variability was not an independent predictor of poor glycemic control in a population aged 65 years or older with diabetes in Medicare Advantage plans. However, mean HbA1c, absolute change in HbA1c level, number of primary care visits, number of specialist visits, and insulin use were statistically significantly associated with poor glycemic control.
Although diabetes care has been improved over time, the magnitude of poor glycemic control in older diabetic patients is high [7,8]. For instance, a study conducted in older Mexican American diabetics aged ≥ 75 years showed 65.1% had HbA1c > 7.0% [25], and 38.4% Malaysian patients aged ≥ 60 years had HbA1c ≥ 8.0% [26]. We found nearly 22% of older adults aged ≥ 65 years with diabetes had poor glycemic control (HbA1c ≤ 9.0%), suggesting that there is room to improve diabetes outcomes. Moreover, different researchers have shown that poor glycemic control of diabetic patients leads to microvascular and macrovascular complications. However, lowering HbA1 concentrations significantly reduces the rate of progression of microvascular complications. A group-level meta-analysis of ACCORD, ADVANCE, and VADT suggested that glucose lowering had a modest (9%) but statistically significant reduction in major cardiovascular disease, primarily nonfatal myocardial infarction outcomes [5].
There are potentially many factors behind the challenges in glycemic control investigation. Healthcare claims databases do not contain some robust information such as body mass index (BMI), blood pressure, and stress management. Robust evidence may require high quality long-term prospective study or randomized cohort data. Recently several studies have examined socio-demographic, behavioral, and treatment related factors behind glycemic control and generated new insights on the potential factors of glycemic outcomes [25-29].
A unique strength of our study was the ability to assess longitudinal HbA1c profile and comprehensive characteristics of patients and link these characteristics to glycemic control in a large retrospective cohort design. To our knowledge, ours is the first such study. We found that the proportion of patients who had poor glycemic control increased with increasing quintiles of HbA1c variability and increasing quintiles of HbA1c variability are associated with both increased mean HbA1c and absolute change in HbA1c. Similar to the other study on HbA1c variability and adherence to antidiabetic medications [17], we didn't find significant association between HbA1c variability and poor glycemic control in the full adjusted model. This could have been the result of the correlation of mean HbA1c and HbA1c variability. In the univariate analysis, there was a moderately strong association between HbA1c variability and poor glycemic control. However, no association was found after adjustment by mean HbA1c and other co-variables. Mean HbA1c was the most significant predictor and absolute change in HbA1c was marginally associated with poor glycemic control.
In this study, patients who visited their primary care physicians (PCPs) more frequently or visited their specialists less were less likely to have poor glycemic control. More specialist visits may indicate that the diabetes is more severe and/or patients are more complex with multiple comorbidities. The patients who visited their PCPs more often may have the opportunity for more intensive monitoring of their HbA1c. PCPs might have been more able to focus on a patient's diabetes management because other medical problems were being addressed by specialist providers. Similar to other reports, we found that patients who received insulin treatment [30,31] or with chronic kidney disease [32] had higher likelihood of poor glycemic control.
Additional factors associated with poor glycemic control in older adults, including longer duration of diabetes [30,31], lower education level [25,30], ethnic variation [25,33], and lower physical activity [31,34], were not measured in this study. We did include specific diagnoses that often co-occur with diabetes, or that may affect glycemic control, such as obesity, hypertension, hyperlipidemia, stroke, anxiety, depression, ischemic heart disease, and diabetes related complications, such as retinopathy, neuropathy, and nephropathy in this study. We found that all these diseases were not associated with poor glycemic control; some of these results are inconsistent with previous research that showed that obesity [29], depression [35], anxiety [36], diabetic retinopathy [29], diabetic neuropathy [25,29], and diabetic nephropathy [29] are associated with poor glycemic control.
Limitations
Our study had several limitations. First, this study is a retrospective observational study, and we do not know the reasons for having HbA1c measured or the reasons behind the changes in HbA1c. Most likely, the previously measured HbA1c level and the clinical conditions of the patient will have been influenced when a new measurement was made. Those who did not have a measurement in MY2013 or those who did not have at least 3 measurements have been excluded in this cohort. Second, the healthcare claims database that was analyzed had no information of diabetic duration, severity of disease, and lifestyles, such as diet, exercise and drinking habits, which may influence outcomes. Third, we obtained household income and education level from US census data at a zipcode level. This data may be not accurate at an individual level. Fourth, our study cohort consists of Medicare beneficiaries at a single managed health care system; although the sample size is relative big, the findings may not apply to other setting. Fifth, the use of ICD-9 diagnosis codes is not as rigorous as formal clinical assessments and may underrepresent certain conditions. Finally, the analyses may not have captured all variables as previously discussed that may potentially impact glycemic control.
Conclusion
This real-world retrospective study has shown that the rate of poor glycemic control among older adults with diabetes is still high and HbA1c variability is not a robust indicator of poor glycemic control; however, mean HbA1c, absolute change in HbA1c level, number of primary care visits, number of specialist visits, and insulin use are significantly associated with poor glycemic control. Further studies are needed to explore this finding and to determine the association of other variables with glycemic control. Future research should use all available information to develop a reliable predictive tool to identify at-risk patients and deliver appropriate interventions to target population.
Acknowledgements
The authors thank Howard Chapman, Keith A Layton, Ibrul Chowdhury, and Feiyan Chen of Clinical Analytics @Independence Blue Cross, Philadelphia, for their assistance in data collection, analysis, and review.
Financial Disclosures
The authors declare that they have no relevant financial relationships to disclose.
Conflict of Interest
The authors have no conflicts of interest to disclose.
Funding/Support
This study was funded by Independence Blue Cross, which is an independent licensee of the Blue Cross and Blue Shield Association. All of the authors were employed by Independence Blue Cross during the course of the study.
References
-
Lozano R, Naghavi M, Foreman K, Lim S, Shibuya K, et al. (2012) Global and regional mortality from 235 causes of death for 20 age groups in 1990 and 2010: a systematic analysis for the Global Burden of Disease Study 2010. Lancet 380: 2095-2128.
-
King H, Aubert R, Herman W (1998) Global burden of diabetes, 1995-2025: prevalence, numerical estimates, and projections. Diabetes Care 21: 1414-1431.
-
The Diabetes Control and Complications Trial Research Group (1993) The Effect of Intensive Treatment of Diabetes on the Development and Progression of Long-Term Complications in Insulin-Dependent Diabetes Mellitus. N Engl J Med 329: 977-986.
-
UK Prospective Diabetes Study (UKPDS) Group (1998) Intensive Blood-Glucose Control with Sulphonylureas or Insulin Compared with Conventional Treatment and Risk of Complications in Patients with Type 2 Diabetes (UKPDS 33). Lancet 352: 837-853.
-
American Diabetes Association (2015) Glycemic Targets: In Standards of Medical Care in Diabetes-2015. Diabetes Care 38: s33-40.
-
Wagner EH, Sandhu N, Newton KM, McCulloch DK, Ramsey SD, et al. (2001) Effect of improved glycemic control on health care costs and utilization. JAMA 285: 182-189.
-
Tan E, Yang W, Pang B, Dai M, Loh FE, et al. (2015) Geographic Variation in Antidiabetic Agent Adherence and Glycemic Control Among Patients with Type 2 Diabetes. J Manag Care Spec Pharm 21: 1195-1202.
-
Ali MK, Bullard KM, Saaddine JB, Cowie CC, Imperatore G, et al. (2013) Achievement of goals in U.S. diabetes care, 1999-2010. N Engl J Med 368: 1613-1624.
-
Sprague L (2015) The Star Rating System and Medicare Advantage Plans. Issue Brief Natl Health Policy Forum 1-10.
-
https://www.cms.gov/Medicare/Prescription-Drug-Coverage/PrescriptionDrugCovGenIn/Downloads/2016-Technical-Notes-Preview-1-v2015_08_05.pdf.
-
Jung HS (2015) Clinical Implications of Glucose Variability: Chronic Complications of Diabetes. Endocrinol Metab (Seoul) 30: 167-174.
-
Rodríguez-Segade S, Rodríguez J, García López JM, Casanueva FF, Camiña F (2012) Intrapersonal HbA(1c) variability and the risk of progression of nephropathy in patients with Type 2 diabetes. Diabet Med 29: 1562-1566.
-
Penno G, Solini A, Bonora E, Fondelli C, Orsi E, et al. (2013) HbA1c variability as an independent correlate of nephropathy, but not retinopathy, in patients with type 2 diabetes: the Renal Insufficiency And Cardiovascular Events (RIACE) Italian Multicenter Study. Diabetes Care 36: 2301-2310.
-
Zoungas S, Chalmers J, Ninomiya T, Li Q, Cooper ME, et al. (2012) Association of HbA1c levels with vascular complications and death in patients with type 2 diabetes: evidence of glycaemic thresholds. Diabetologia 55: 636-643.
-
Currie CJ, Peters JR, Tynan A, Evans M, Heine RJ, et al. (2010) Survival as a function of HbA(1c) in people with type 2 diabetes: a retrospective cohort study. Lancet 375: 481-489.
-
Skriver MV, Sandbaek A, Kristensen JK, Stovring H (2015) Relationship of HbA1c variability, absolute changes in HbA1c, and all-cause mortality in type 2 diabetes: a Danish population-based prospective observational study. BMJ Open Diabetes Res Care 2.
-
Ramachandran A, Winter M, Mann DM (2015) Association of visit-to-visit variability of hemoglobin A1c and medication adherence. J Manag Care Spec Pharm 21: 229-237.
-
Hedis and performance measurement. National Committee for Quality Assurance website.
-
http://www.ncqa.org/portals/0/PolicyUpdates/HEDIS%20Technical%20Updates/09_CDC_Spec.pdf.
-
Charlson ME, Charlson RE, Peterson JC, Marinopoulos SS, Briggs WM, et al. (2008) The Charlson comorbidity index is adapted to predict costs of chronic disease in primary care patients. J Clin Epidemiol 61: 1234-1240.
-
Deyo RA, Cherkin DC, Ciol MA (1992) Adapting a clinical comorbidity index for use with ICD-9-CM administrative databases. J Clin Epidemiol 45: 613-619.
-
(2014) Sightlines DxCG Risk Solutions: Concepts and Methodologies Guide for UNIX. Waltham MA: Verisk Health, Inc.
-
Hess LM, Raebel MA, Conner DA, Malone DC (2006) Measurement of adherence in pharmacy administrative databases: a proposal for standard definitions and preferred measures. Ann Pharmacother 40: 1280-1288.
-
Osterberg L, Blaschke T (2005) Adherence to medication. N Engl J Med 353: 487-497.
-
Otiniano ME, AI Snih S, Goodwin JS, Ray L, AlGhatrif M, et al. (2012) Factors associated with poor glycemic control in older Mexican American diabetics aged 75 years and older. J Diabetes Complications 26: 181-186.
-
Sazlina SG, Mastura I, Cheong AT, Bujang Mohamad A, Jamaiyah H, et al. (2015) Predictors of poor glycaemic control in older patients with type 2 diabetes mellitus. Singapore Med J 56: 284-290.
-
Chiu CJ, Wray LA (2010) Factors predicting glycemic control in middle-aged and older adults with type 2 diabetes. Prev Chronic Dis 7: A08.
-
McAdam-Marx C, Bellows BK, Unni S, Wygant G, Mukherjee J, et al. (2014) Impact of adherence and weight loss on glycemic control in patients with type 2 diabetes: Cohort analyses of integrated medical record, pharmacy claims, and patient-reported data. J Manag Care Spec Pharm 20: 691-700.
-
Bae JP, Lage MJ, Mo D, Nelson DR, Hoogwerf BJ (2016) Obesity and glycemic control in patients with diabetes mellitus: Analysis of physician electronic health records in the US from 2009-2011. J Diabetes Complications 30: 212-220.
-
Goudswaard AN, Stolk RP, Zuithoff P, Rutten GE (2004) Patient characteristics do not predict poor glycaemic control in type 2 diabetes patients treated in primary care. Eur J Epidemiol 19: 541-545.
-
LeBlanc ES, Rosales AG, Kachroo S, Mukherjee J, Funk KL, et al. (2015) Do patient or provider characteristics impact management of diabetes? Am J Manag Care 21: 597-606.
-
Bash LD, Selvin E, Steffes M, Coresh J, Astor BC (2008) Poor glycemic control in diabetes and the risk of incident chronic kidney disease even in the absence of albuminuria and retinopathy: Atherosclerosis Risk in Communities (ARIC) Study. Arch Intern Med 168: 2440-2447.
-
Egede LE, Gebregziabher M, Hunt KJ, Axon RN, Echols C, et al. (2011) Regional, geographic, and racial/ethnic variation in glycemic control in a national sample of veterans with diabetes. Diabetes Care 34: 938-943.
-
Gregg EW, Chen H, Wagenknecht LE, Clark JM, Delahanty LM, et al. (2012) Association of an intensive lifestyle intervention with remission of type 2 diabetes. JAMA 308: 2489-2496.
-
Papelbaum M, Moreira RO, Coutinho W, Kupfer R, Zagury L, et al. (2011) Depression, glycemic control and type 2 diabetes. Diabetol Metab Syndr 3: 26.
-
Anderson RJ, Grigsby AB, Freedland KE, de Groot M, McGill JB, et al. (2002) Anxiety and poor glycemic control: a meta-analytic review of the literature. Int J Psychiatry Med 32: 235-247.
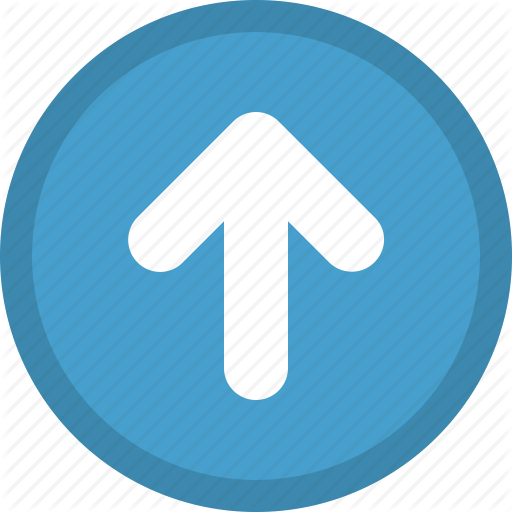